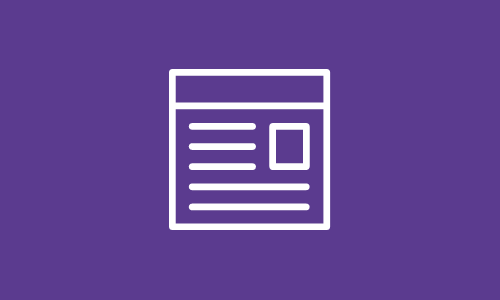
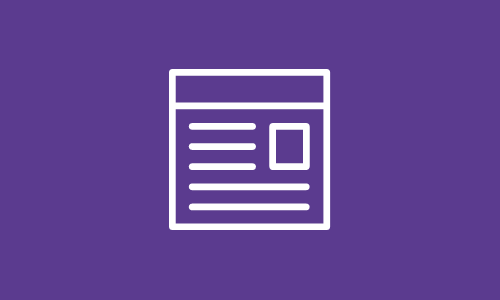
School officials regularly use school-aggregate test scores to monitor school performance and make policy decisions. In this report, RAND researchers investigate one specific issue that may contaminate utilization of COVID-19–era school-aggregate scores and result in faulty comparisons with historical and other proximal aggregate scores: changes in school composition over time. To investigate this issue, they examine data from NWEA’s MAP Growth assessments, interim assessments used by states and districts during the 2020–2021 school year.
By: Jonathan Schweig, Megan Kuhfeld, Andrew McEachin, Melissa Diliberti, Louis Mariano
Topics: Measurement & scaling, COVID-19 & schools
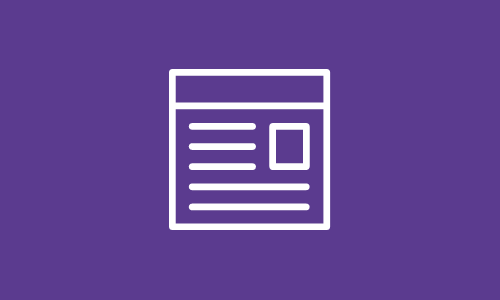
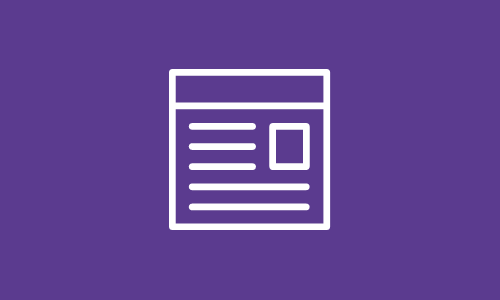
Supporting students with disabilities throughout the year
Students with disabilities lose even more ground than peers during summer and other interruptions in their learning—but they don’t need to. Data point to a need for services that extend beyond the school year.
By: Elizabeth Barker, Angela Johnson
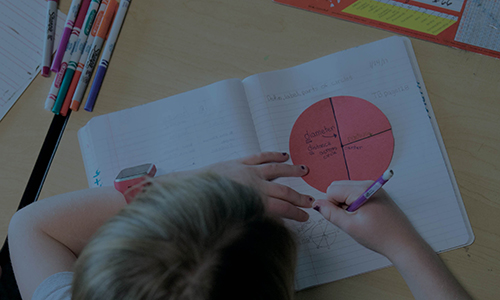
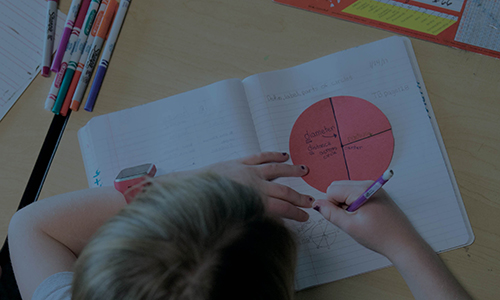
To what extent has the COVID-19 pandemic affected student achievement and growth in reading and math, and which students have been most affected? Using data from 6 million students in grades 3-8 who took MAP Growth assessments in reading and math, this brief examines how gains across the pandemic (fall 2019 to fall 2021) and student achievement in fall 2021 compare to pre-pandemic trends. This research provides insight to leaders working to support recovery.
By: Karyn Lewis, Megan Kuhfeld
Topics: COVID-19 & schools, Equity, Growth modeling
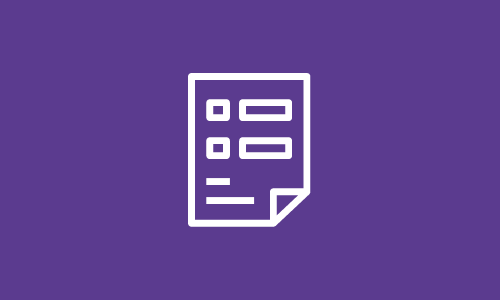
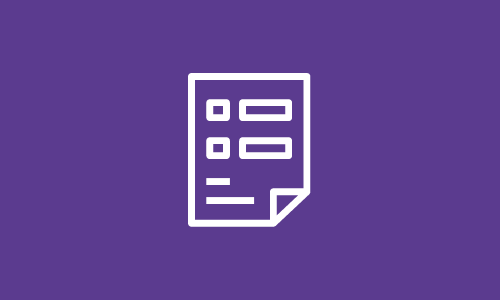
The purpose of this technical appendix is to share more detailed results and to describe more fully the sample and methods used in the research included in the brief, Learning during COVID-19: An update on student achievement and growth at the start of the 2021-22 school year. We investigated two research questions:
- How does student achievement in fall 2021 compare to pre-pandemic levels (namely fall 2019)?
- How did academic gains between fall 2019 and fall 2021 compare to normative growth expectations?
By: Megan Kuhfeld, Karyn Lewis
Topics: COVID-19 & schools, Equity, Growth modeling
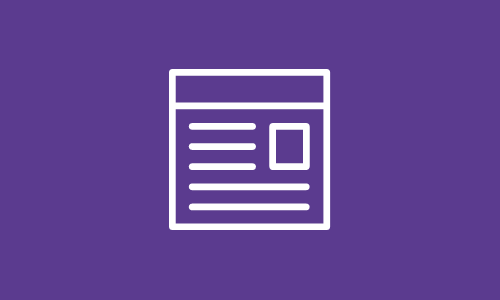
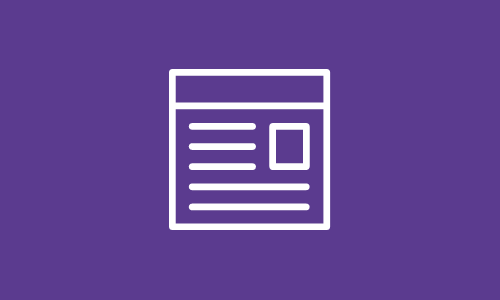
Examining the performance of the trifactor model for multiple raters
Using simulations, this study examined the “trifactor model,” a recent model developed to address rater disagreement.
By: James Soland, Megan Kuhfeld
Topics: Measurement & scaling
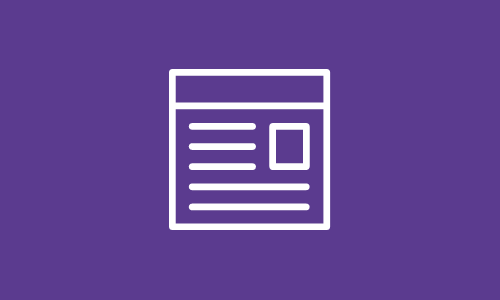
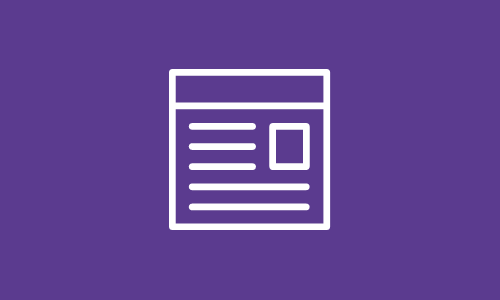
Achievement growth in K-8 Catholic schools using NWEA data
Using a national sample of kindergarten to eighth grade students from Catholic and public schools who took MAP Growth assessments, we examine achievement growth over time between sectors.
By: Julie Dallavis, Megan Kuhfeld, Beth Tarasawa, Stephen Ponisciak
Topics: Early learning, Middle school
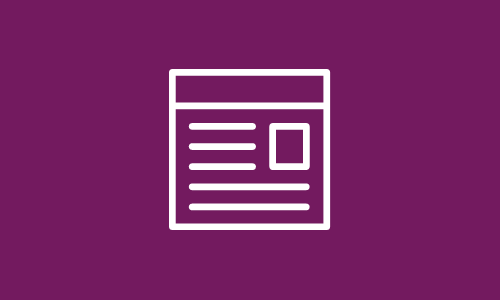
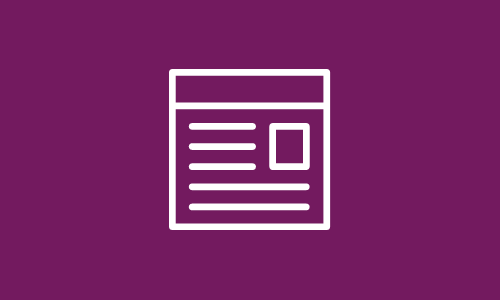
BFpack: Flexible Bayes factor testing of scientific theories in R
In this paper we present a new R package called BFpack that contains functions for Bayes factor hypothesis testing for the many common testing problems. The software includes novel tools for (i) Bayesian exploratory testing (e.g., zero vs positive vs negative effects), (ii) Bayesian confirmatory testing (competing hypotheses with equality and/or order constraints), (iii) common statistical analyses, such as linear regression, generalized linear models, (multivariate) analysis of (co)variance, correlation analysis, and random intercept models, (iv) using default priors, and (v) while allowing data to contain missing observations that are missing at random.
By: Joris Mulder, Donald Williams, Xin Gu, Andrew Tomarken, Florian Böing-Messing, Anton Olsson-Collentine, Marlyne Meijerink-Bosman, Janosch Menke, Robbie van Aert, Jean-Paul Fox, Herbert Hoijtink, Yves Rosseel, Eric-Jan Wagenmakers, Caspar van Lissa
Topics: Measurement & scaling