Journal article
Predicting the difficulty and response time of multiple choice questions using transfer learning
2020
15th Workshop on Innovative Use of NLP for Building Educational Applications
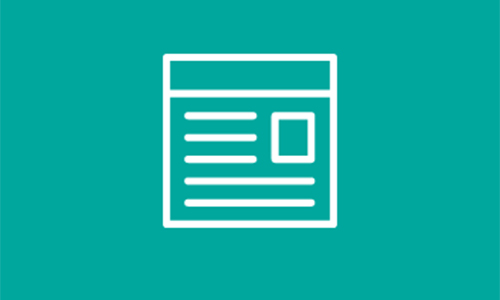
Abstract
This paper reports on whether transfer learning can improve the prediction of the difficulty and response time parameters for ā 18,000 multiple-choice questions from a high-stakes medical exam. The type of the signal that bestpredicts difficulty and response time is also explored, both in terms of representation abstraction and item component used as input (e.g., whole item, answer options only, etc.). Theresults indicate that, for our sample, transferlearning can improve the prediction of item difficulty when response time is used as an auxiliary task but not the other way around. In addition, difficulty was best predicted using signal from the item stem (the description of theclinical case), while all parts of the item were important for predicting the response time.
See MoreThis article was published outside of NWEA. The full text can be found at the link above.